The sheer volume and diversity of data have transformed the way organizations operate. Managing and making sense of this diverse dataset requires tools and approaches that empower businesses across different departments to derive insights independently. To effectively harness the wealth of minute details buried within the datasets, the concept of self-service analytics has emerged as a transformative force. Let’s delve into the intricacies of the same, exploring the fundamental principles, key features, and the undeniable impact it has on the BI landscape.
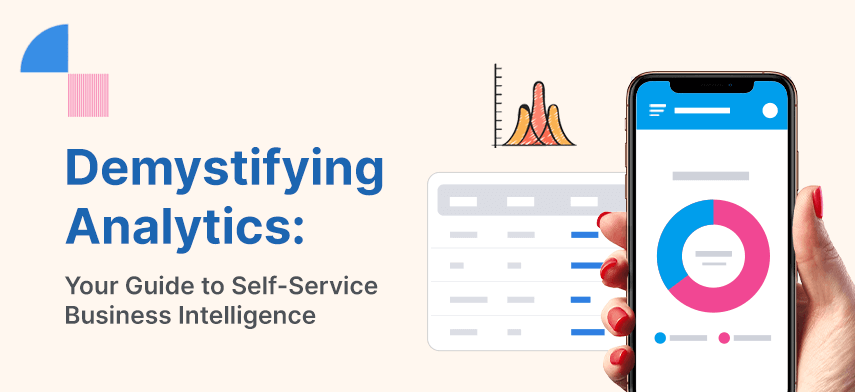
What is Self-service Analytics?
Self-service analytics refers to a modern-day approach to business intelligence that empowers line-of-business professionals to conduct data queries and create reports without relying on IT or data experts for support. This allows them to access and analyze business data to extract meaningful insights and make data-driven decisions independently.
Unlike traditional methods where specialized data teams or analysts are primarily responsible for interpreting data, Self Service Business Intelligence (SSBI) and analytics provide tools and accessible insights directly to employees. Empowering non-technical users with BI means fostering a data-driven culture where users can confidently navigate, analyze, and derive valuable information. This approach bridges the gap between technical complexity and user-friendly empowerment in the realm of business intelligence.
Moreover, implementing self-service BI for small businesses can democratize data access and analysis, enabling decision-makers at all levels to make informed choices. Now, let’s explore some self service BI use cases across various departments.
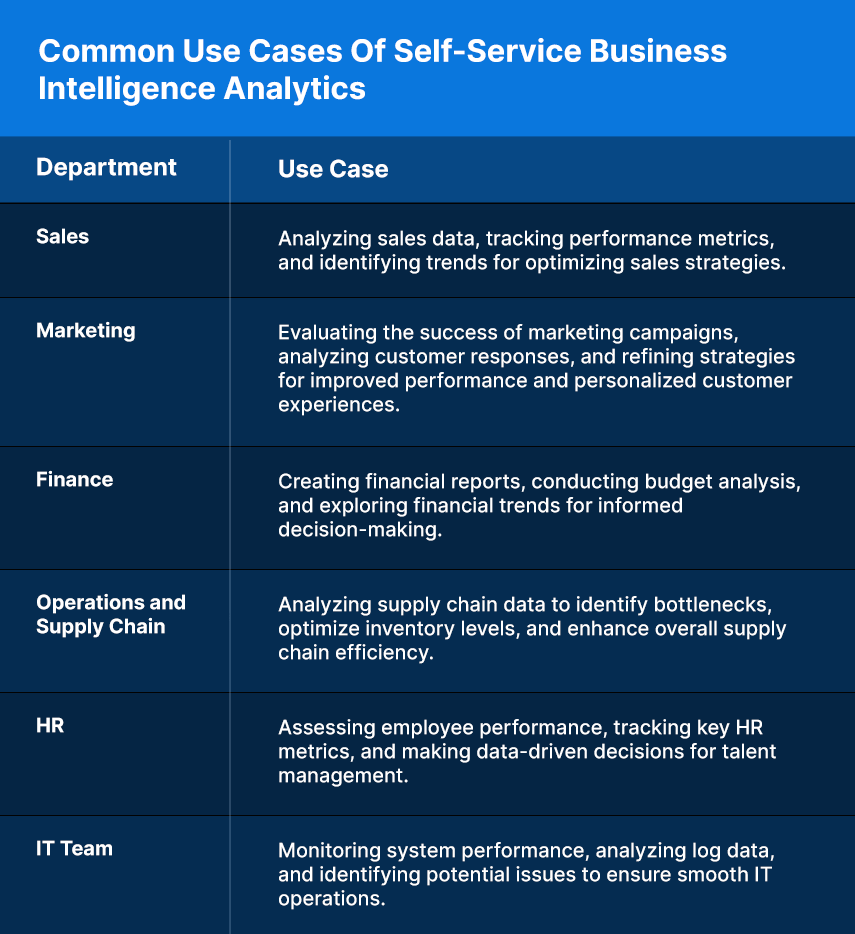
Key Components and Features
The key components of self-service business intelligence analytics encompass a range of features and functionalities designed to be approachable, featuring basic analytic capabilities for users. Here are the key components:
An easy-to-use interface
Some user-friendly analytics tools are specifically designed to empower business users, regardless of their technical expertise, by simplifying the data analysis process.
- Intuitive dashboards provide visual analytics of data. These user-centric dashboards are designed to make it easy for individuals with varying technical backgrounds to navigate and understand.
- With drag-and-drop functionality, users can easily select and arrange diverse data elements. They can choose from a variety of charts, heatmaps, graphs, scatter plots, and other visual elements to represent data in a way that is most meaningful to them and their audience.
- Users can see real-time or near-real-time data updates when they construct any report with the WYSIWYG editing features.
- Pre-built templates help users streamline common data analysis tasks.
- Customization tools provide flexibility in adjusting visual elements to match branding or reporting standards. Furthermore, interactive elements, such as tooltips, filters, and parameters, enhance engagement and informativeness.
Data Preparedness, Accessibility and Integration
Smart capabilities of self-service analytics tools empower users to navigate data complexities and create data discovery with ease. As a result, data analytics workflows become more efficient and accurate.
- Tools provide data connectors to various sources, ensuring unified integration with databases and cloud services.
- Users can cleanse, modify, and restructure business data with data-wrangling tools. It helps handle activities like eliminating duplicates and filling in missing values, making sure the data is ready for analysis.
- Users can interactively query datasets, enabling filtering, sorting, and data transformation.
- Collaboration features enable users to work together in real time, sharing data insights, dashboards, and analyses.
- Users can merge various data origins like spreadsheets and cloud storage databases using self service BI software.
- Automated ETL processes boost effectiveness by automating extraction, transformation, and loading operations, which minimize manual work and assure data coherence.
- Data profiling feature also plays a crucial role in providing insights into data characteristics like distribution and types, aiding users in identifying and addressing data quality issues for well-informed decision-making.
Others:
- Advanced Analytics and Predictive Modeling: Users can apply machine learning algorithms and statistical models without extensive technical expertise.
- Security in Self Service BI: To ensure data security, these tools incorporate role-based access controls. These controls restrict access to sensitive information based on the roles.
- Conversational Analytics: Integration with NLP allows users to interact using natural language queries, enhancing accessibility.
- Mobile Accessibility: Designed with responsive interfaces, these tools offer flexibility for users to access and interact with data using mobile devices.
- Training and Support Resources: To facilitate user adoption, these tools provide training resources and support materials, aiding users in navigating and utilizing the tool effectively.
Understanding these key components is essential for organizations looking to implement and leverage self service business intelligence and analytics effectively.

Necessity of Self-service Analytics
The adoption of self-service BI is necessitated by the evolving nature of the current data landscape, characterized by:
Data Abundance
The modern world is generating vast amounts of data from diverse sources, including social media, IoT devices, and online transactions. This data requires a more agile and inclusive approach to analysis.
Real-time Decision-Making
Businesses operate in dynamic and fast-paced environments where delayed decision-making can result in missed opportunities or increased risks. Self service BI solutions enable users to access up-to-date data without delays.
Diverse Data Types
With data being generated in various formats, including unstructured text, images, and videos, self-service analytics tools cater to the need for analyzing diverse data types.
Skill Gap and Resource Optimization
There is often a shortage of skilled data professionals, and traditional data analysis methods may create bottlenecks. Self-service business intelligence and analytics bridge the skill gap by allowing non-technical users to actively participate in the data analysis process.
Business Agility
Businesses need to adapt quickly to changing market conditions, customer preferences, and emerging trends. User-friendly BI tools provide the agility required for users to independently explore and analyze data.
In the early days of data analysis, accessing and interpreting data required specialized skills. However, as technology advanced, the need for democratizing data became apparent. Self-service BI emerged as a response, breaking down the barriers and making data accessible to a broader audience within organizations. Let’s deep dive into the benefits of self service analytics for businesses.
Benefits of Self-service BI
At its core, the user-friendly BI tool analytics breaks barriers between data analysis and the decision-making process, facilitating business growth. The adoption of the new form of BI yields a multitude of benefits for organizations looking to harness the full potential of their data.
Quick data-driven decision making with self service BI
A centralized data hub serves as a focus point of entire information for decision-makers, streamlining hours of inefficient data workflows. It helps users to gain and consolidate collective data insights, analyze trends, and make informed decisions promptly. There is no need for them to wait for a data analyst or any IT team member to provide specific information in response to their data requests. This is important in today’s fast-paced environment.
Increased user adoption among departments
Self service BI, with its user-friendly interfaces, encourages broader adoption. Business users, especially non-technical users, feel empowered to engage with data directly and do ad hoc analysis without the IT team’s support. The result is a culture in which everyone is motivated to leverage data for decision-making and work in an agile environment.
Achieve cost-efficiency
Advanced self-service business intelligence tools enhance cost-efficiency for businesses by reducing reliance on manual labor. Organizations can optimize entire data processing, allocate resources more efficiently, and avoid bottlenecks in the data analysis pipeline.
Time Optimization
In the traditional approach, the data analysis process was time-consuming, demanding assistance from the IT team or any data experts. By using self-service BI, users from different departments can manage daily data-related requests or create reports on their own. This reduces the workload of IT as well as data teams, allowing them to focus on more strategic initiatives and higher-value tasks.
Step-by-Step Process for Implementing Self-Service Analytics
Implementing self-service analytics involves a structured process to ensure successful adoption and utilization within an organization. Below is a step-by-step self service BI implementation guide:
Step 1: Assess business workflows necessitating data-driven insights
Begin by conducting a thorough assessment of your organization’s data sources and workflows that are time-intensive, repetitive, and require up-to-date information. Engage with key stakeholders to identify specific pain points and challenges that a new form BI can address. Define clear and measurable objectives to introduce self-service BI for these tasks.
Step 2: Select the Right Tools
Choose modern, BI self service data analytics tools that align with your requirements. Consider factors such as user-friendliness, scalability, integration capabilities, customizable AI and ML models, embedded APIs, and support for various data sources. The selected tools should empower users across different roles to analyze data effectively and should offer good ROI.
Step 3: Establish Robust Data Governance
Implement strong data governance policies to ensure the quality, security, and compliance of your data. Define roles and responsibilities, set data quality standards, and establish protocols for data sharing. A solid data governance framework is crucial for maintaining the integrity of a self service analytics platform.
Step 4: Provide Training to Users
Invest in comprehensive user training programs to guide how to use the self-service analytics platform for all users. Tailor training sessions to different user groups, IT teams, and data professionals. Ensuring users feel confident and supported enhances adoption rates.
Step 5: Encourage a Data-Driven Culture
Foster a culture that values data-driven decision making. Promote the use of data for insights, and celebrate successes achieved through self-service data analytics. Encourage a mindset where data is seen as a valuable resource.
Step 6: Start a Pilot Program, Iterate and Improve
Initiate a pilot program with a small group of users to test the effectiveness of the selected tools in a real-world scenario. To ensure seamless adoption, self-service analytics should integrate seamlessly into existing workflows. So start by connecting your existing self service business analytics data sources and let your team build their first reports of a few important business functions. Gather feedback from stakeholders, identify areas for improvement, and make necessary adjustments before a full-scale rollout.
Embrace a culture of collaboration and continuous improvement, regularly assessing user feedback and evolving the implementation to meet changing needs.
What is the Future of Self-service Analytics?
Looking ahead, the data landscape promises exciting advancements that will elevate the realm of self-service analytics.
- AI-driven self-service analytics will witness impactful advancements such as AI Data Applications automating analytics with advanced algorithms for real-time anomaly detection and personalized recommendations.
- Large Language Models (LLMs) will revolutionize data interaction. It will enable users to curate, query, and analyze data using natural language interfaces.
- Machine Learning Predictions will equip organizations with accurate forecasting capabilities using historical data in areas like demand prediction, inventory optimization, and fraud detection.
Conclusion
Self-service analytics is a strategic imperative for modern businesses. It empowers non-technical individuals to become active participants in the data-driven decision-making process, promoting agility, accessibility, and innovation. As businesses navigate an era of increasing data complexity, the call to action resonates loud and clear – embrace the potential of self-service analytics. Connect with us to get started with it today!
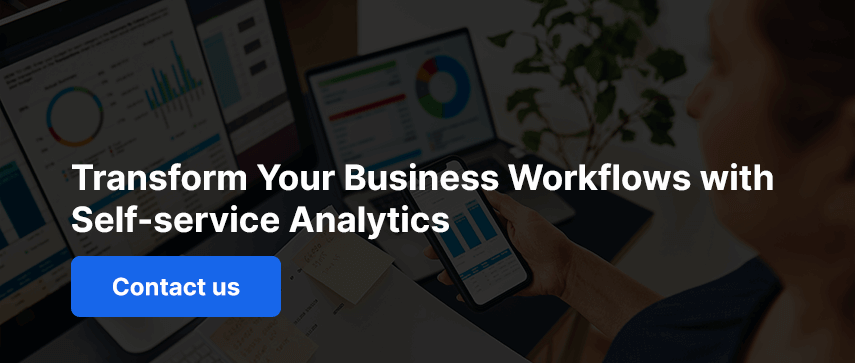
Author's Bio
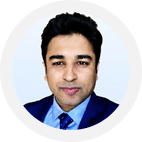
Nitin Lahoti is the Co-Founder and Director at Mobisoft Infotech. He has 15 years of experience in Design, Business Development and Startups. His expertise is in Product Ideation, UX/UI design, Startup consulting and mentoring. He prefers business readings and loves traveling.